top of page
Unlocking Competitive Edge: The Power of RAG AI Agents in Business
May 04, 2025
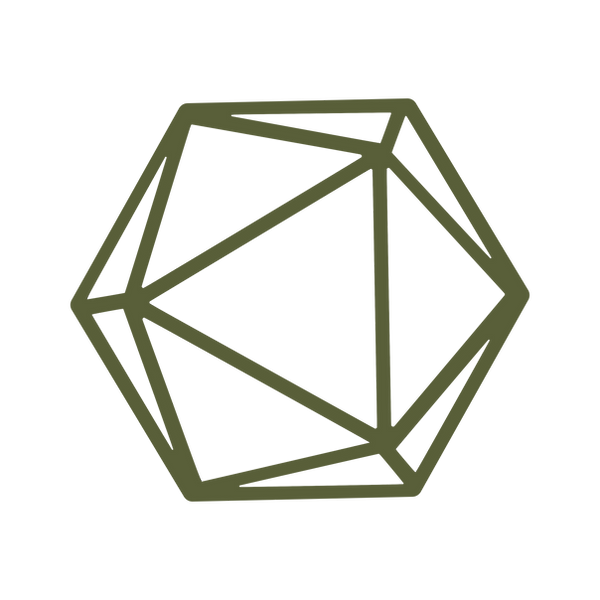
Discover how Retrieval-Augmented Generation (RAG) AI agents are poised to transform the business landscape by merging real-time data retrieval with powerful language processing. This article delves into how RAG technology enhances operational efficiency, fosters informed decision-making, and integrates seamlessly with existing systems, offering a distinct competitive edge. Learn about best practices for implementation, the challenges organizations face, and what the future holds for AI-driven operations. As businesses increasingly adopt these intelligent agents, you won't want to miss insights into optimizing your AI strategy for success. Read on to unlock your organization's potential!
Introduction to RAG AI Agents
Retrieval-Augmented Generation (RAG) AI agents represent a revolutionary approach to artificial intelligence that combines the power of large language models with real-time data retrieval capabilities. These systems enhance AI applications by accessing and incorporating current, relevant information into their responses Google Cloud.
Unlike traditional AI models, RAG agents can maintain up-to-date knowledge by pulling information from authorized data sources, ensuring accuracy and relevance in decision-making processes. This capability significantly reduces the risk of outdated or incorrect information affecting business operations Microsoft.
The technology is particularly valuable for organizations seeking to leverage their existing data infrastructure while maintaining control over information sources. RAG agents can seamlessly integrate with company databases, documentation, and knowledge bases, providing more precise and contextually relevant responses than traditional AI models Coveo.
The Strategic Advantage of RAG in Businesses
In today's data-driven business landscape, RAG is emerging as a game-changing technology that provides organizations with significant competitive advantages. By combining the power of large language models (LLMs) with precise information retrieval systems, RAG enables businesses to make more informed decisions and streamline operations AWS.
Cost efficiency represents another crucial advantage. Unlike traditional AI implementations that require expensive retraining to incorporate new information, RAG enables businesses to seamlessly integrate fresh data into their AI systems without significant additional investment TapClicks.
RAG enhances enterprise knowledge management by connecting AI systems directly to internal knowledge bases and documentation. This integration enables more accurate, context-aware responses in customer service, decision support, and operational workflows IngestAI.
Implementing RAG AI Agents: Best Practices
Recent studies show that organizations implementing RAG systems have seen up to 35% improvement in operational efficiency AdaSci. Successful implementation requires careful attention to data foundation preparation, including establishing a robust knowledge base and implementing effective data chunking strategies IntelliArts.
Context-aware implementation and performance optimization are crucial factors. Organizations should design systems that provide transparency in output generation and implement efficient vector databases for faster retrieval Nexla.
Quality assurance and monitoring play vital roles in successful RAG implementation. This includes implementing robust testing protocols, establishing clear metrics for measuring system performance, and regular auditing of responses Evidently AI.
Overcoming Challenges: Navigating RAG Implementation
Data integration emerges as a primary hurdle, where organizations must effectively consolidate information from various internal systems. Quality control poses another significant challenge, particularly in maintaining response accuracy and relevance Denser.ai.
Security and compliance considerations often create implementation bottlenecks. Successful RAG adoption requires careful attention to data privacy, especially when handling sensitive business information. Organizations should prioritize implementing strong authentication mechanisms and maintaining clear audit trails of data usage Intelliarts.
The Future of Business with RAG AI Agents
According to industry forecasts, 25% of enterprises using generative AI will deploy AI agents by 2025, with adoption reaching 50% by 2027 Analytics Vidhya. Enhanced autonomy will be a primary driver, with AI agents becoming increasingly capable of operating independently and making complex decisions with minimal human oversight.
Organizations will transition from single-agent solutions to multi-agent teams handling complex business scenarios, while AI copilots will become standardized tools across various business functions CIO.
ScaleWize AI helps organizations navigate these challenges by providing customized RAG AI solutions that align with specific business needs. Our expertise in implementing and optimizing RAG systems ensures smooth integration, maximum efficiency, and measurable business impact. With our support, businesses can confidently embrace the future of AI-driven operations while maintaining focus on their core objectives.
Sources
- AdaSci - Why Do Enterprises Love RAG?
- Analytics Vidhya - AI Agent Trends
- AWS - What is Retrieval-Augmented Generation?
- Google Cloud - Retrieval-Augmented Generation Use Cases
- Coveo - Retrieval-Augmented Generation Benefits
- Denser.ai - Enterprise RAG Implementation
- Evidently AI - RAG Examples
- IngestAI - RAG in Enterprise AI
- Intelliarts - Enterprise RAG System Best Practices
- Microsoft - 5 Key Features and Benefits of RAG
- Nexla - Retrieval-Augmented Generation
- TapClicks - RAG Benefits for Business
- CIO - The Future of AI Agents
bottom of page